A University of Washington School of Public Health researcher has adapted a text-mining tool to identify new patterns in the electronic health records (EHR) of sepsis patients. The methodology could lead to more precise treatment of patients with this life-threatening response to infection.
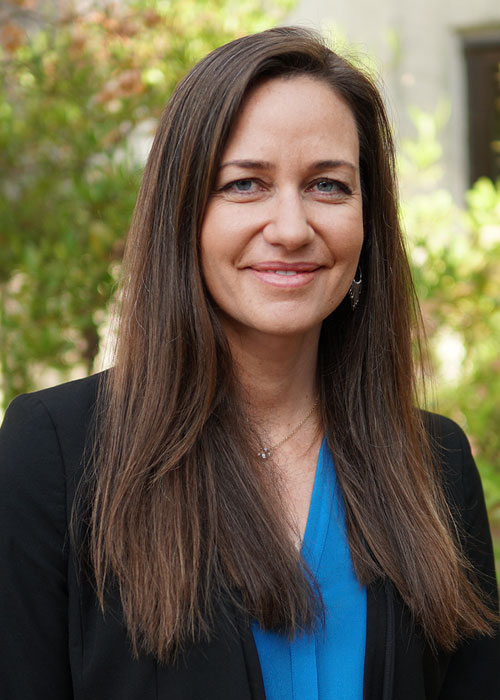
Alison Fohner, assistant professor of epidemiology, led a project at Kaiser Permanente Northern California Division of Research, using a machine learning process to group EHR terms that commonly show up together within the same patients’ health records in early hospitalization. Findings were published July 17 in The Journal of the American Medical Informatics Association.
“The possibilities in this space are pretty huge,” Fohner says. “Text-mining methods were originally designed to uncover meaning in free-form writing like blog posts, emails and social science research, but the analytical capabilities of text mining are immensely useful for other types of data as well.” Data from an integrated health-care system like Kaiser Permanente are well-suited for adapting these outside methods to novel applications, she says, because they are extremely detailed and comprehensive.
Over the last 50 years, there have been no new treatments for sepsis, which afflicts 25 million people annually. The extreme diversity in causes and symptoms of the disease has thwarted attempts to target treatments to particular subgroups of patients, leaving antibiotics and fluids as the universal standard of care. The lack of variation in treatment frustrates researchers like Fohner, who believe groups with shared treatment needs are hidden beneath complicated medical profiles. Identifying such groups could allow us to treat sepsis cases more like cancer, with unique treatment plans based on established disease types.
Previous attempts to group sepsis patients have focused on defining the condition by single labels, like severity or site of infection. Unlike for conditions like cancer, where subcategories of the disease guide vastly different prognoses and treatments, labels to differentiate sepsis patients have not led to meaningfully different treatment options. EHR data, on the other hand, contain all treatments and procedures a person undergoes, and therefore, capture multiple dimensions of their disease and underlying conditions. The machine-generated groupings based on these data can reveal previously unidentified trends among patients.
A process known as ‘precision phenotyping’
Fohner refers to this as precision phenotyping. Much as precision medicine seeks to tailor treatments based on complex genetic patterns, precision phenotyping assumes that diagnoses should also be based around the breadth of data available on each patient, even if the significance of each piece of that data is not fully understood.
The study found a pattern of delayed treatment among patients who presented without obvious symptoms of infection. These patients, Fohner says, should be a focus for improved monitoring and diagnostics. The analysis also suggested that hospital performance evaluations must account for subgroups of patients, such as dialysis and heart failure patients, who are given lower fluid levels because of their sensitivity to this treatment.
The EHR-based groups can also be used to better understand patient responses to treatment in past and future clinical trials. Drugs that failed to show broad benefits across all sepsis patients may prove effective within subsets of patients defined using the machine-learning approach. Fohner asks, “If we apply our categories to clinical trial data that’s been collected already, can we uncover subsets of patients who will do very well on these medications? For 50 years, we’ve been developing new therapies and none of them have really worked on a wholesale level, but we still have the data.” She would like to see these data revisited in light of researchers’ improved ability to categorize these patients.
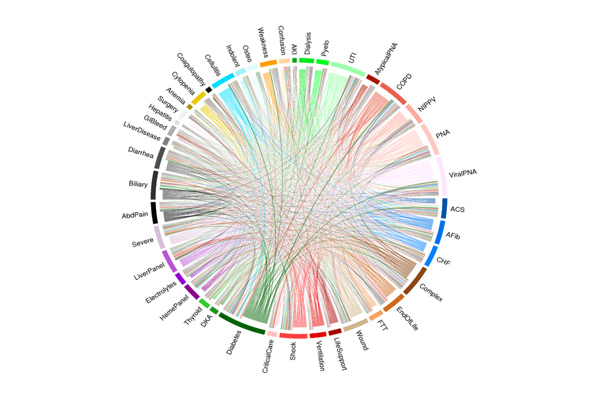
Electronic health records could someday lead to personalized prognoses
While the results of this study confirmed the extreme diversity in sepsis presentation, the very distinct trends researchers had hoped to see in the EHR data remain elusive. Fohner believes future categorization methods could be improved by the inclusion of other patient characteristics, like genetics and biological measures, which may not be well-captured within their current algorithm.
She also imagines that a similar breakdown of patients’ EHR could someday be used to generate personalized prognoses and treatments. But such decision-support metrics, she says, must be thoughtfully integrated to avoid overwhelming the system and to ensure fair and equitable access.
Fohner is a graduate of the UW’s Public Health Genetics program, for which she now teaches, and an adjunct researcher for Kaiser. Co-authors from Kaiser Permanente’s Northern California Division of Research are John Greene, Brian Lawson, Patricia Kipnis, Gabriel Escobar, and Vincent Liu. Co-author Jonathan Chen is an assistant professor of biomedical informatics at Stanford University.